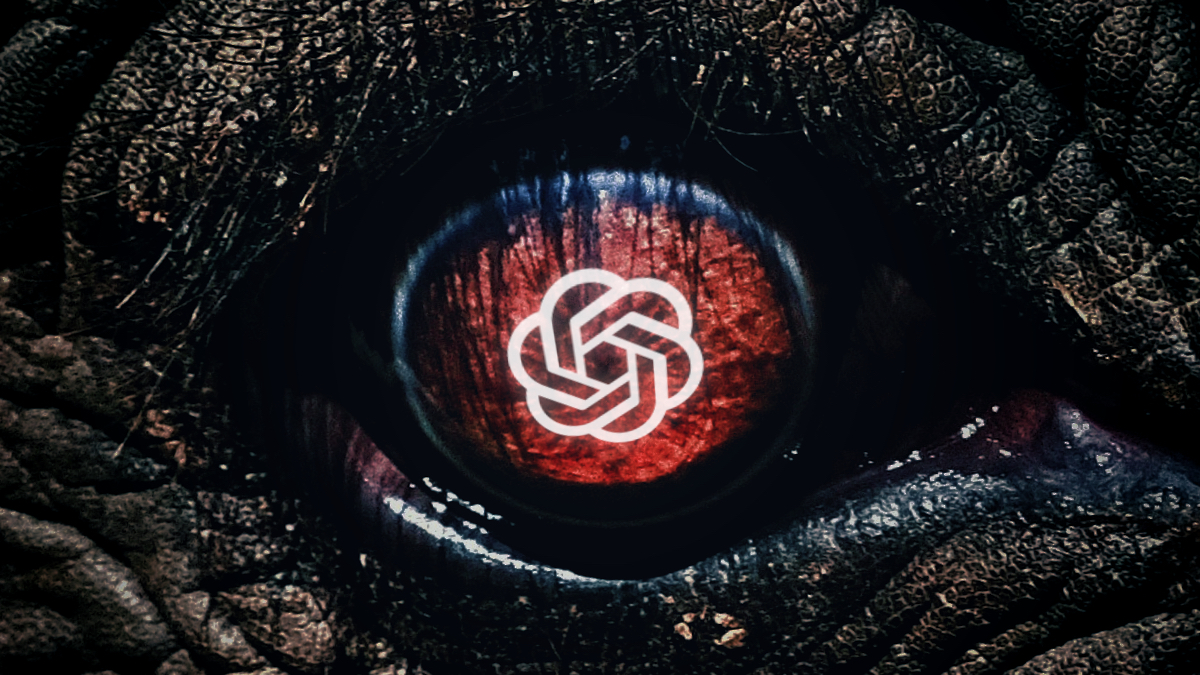
Image by Unsplash, OpenAI (Public Domain) / Montage by Robert Handrow.
-
Introduction
Every couple of months a new AI trend or rather hype captures the news headlines. Yesterday, it was image recognition. Today, we are discussing large language models (LLMs). Tomorrow, who knows. But let’s focus on today’s topic. Most articles about LLMs feature worries about which professions might be (the first in the line to be) replaced by tools such as ChatGPT, while other issues often fly under the radar, at least the radar of the European authorities. One such question is regarding data sets the models are trained on. Are they protected by the GDPR and intellectual property laws? To which extent and when can these data sets then be used to train the models? And what actually occurs when the models ingest data? There has already been some discussion concerning models being trained on copyrighted material without proper licenses. These claims are often being swept under the carpet because the models ‘don’t actually reproduce the works’, however, a team of scientist from Berkely got GPT3 to produce an entire page of the Harry Potter and the Philosopher’s Stone, so J.K. Rowling may beg to differ. And the topic is just starting to heat up when personal data contained in the data sets is concerned, with Italian DPA (‘Garante’) leading the way towards stronger privacy demands imposed on the companies offering the LLMs.
On the other hand, we must also think about the results that these models produce. For instance, GPT3 often confidently offers responses without having any regard if those answers are actually correct. Mixing correct and incorrect data, while providing the answers with the same level of confidence, seems particularly dangerous when thinking about the potential for creating fake news. For instance, in response to a query GPT2 has generated an article about an actual murder of a woman in 2013 and attributed it to an Orlando nightclub shooting victim from 2016. Meta’s BlenderBot characterized a prominent Dutch politician, international policy director at Stanford University’s Cyber Policy Center and an international policy fellow at Stanford’s Institute for Human-Centered Artificial Intelligence, Maria Renske Schaake, as a terrorist. And on a different occasion ChatGPT falsely accused Jonathan Turley, a US law professor, of sexual harassment, even citing a non-existent news article as a source. Offering false information on a murder may not be so damaging if it concerns a person who is obviously not the murderer. However, when bots start flagging active (living) politicians as terrorists or law professors as sex offenders of their own accord, is where we enter the real danger zone. And a disclaimer on GPT3’s Homepage or under the ChatGPT’s text box doesn’t ‘cut it’ as a solution to this problem. This point was also to a certain extent addressed in Garante’s temporary ban imposed on ChatGPT. Their conclusion was that since inaccurate personal data is produced by the model, inaccurate data was necessarily processed in the training. However, one should not oversimplify the accuracy problem and it is very important to differentiate between problems of input as opposed to problems of output. Anyway, to keep things short, in this article we will focus solely on the problem of data ingestion. That is on the question of what is fed into and remains in the model after the data was processed as well as can this be lawful under the current legal framework, while leaving AI ‘hallucinations’ open for further discussion.
-
GPT3's Impact on Personal Data: A Tale of Two Opposing Goals
When talking about large language models such as GPT3, the trade-off for increasing their performance unfortunately appears to be rather clear. The bigger the model, that is the more data it ingests, the better results it produces. This should not come as a surprise, since these models are essentially statistically driven prediction models. They analyze existing data sets (read: textual documents) and make predictions for most probable and therefore best suited textual responses based on their analyses. Therefore, the more data they have access to, the more accurate predictions they can make. This is true because then they have a larger number of parameters, which is directly correlated with more training points being memorized. When this is combined with other advantages associated with LLMs, such as faster convergence, better utilization of available compute and smaller compression errors, the argument for ‘going large’ seems pretty straightforward. On the other hand, large models ingesting a lot of data also memorize a lot more data. Although memorization can occur even after just 33 inclusions in a given training data set, this means that a significantly larger proportion of data will reach this threshold when big models are in question. Simply because they ingest more data. This in turn results in a significant risk of decreased privacy for individuals whose data may be included in the training sets.
Now, various attempts have been made to try and respond to this particular trade off, but weighing privacy against accuracy is a difficult task in several aspects. Primarily because data minimisation, purpose limitation and fairness, are all data protection principles, but so is accuracy. What must not be neglected in this respect, is the fact that AI systems, thus not excluding GPT3, increasingly have an impact on (still predominantly human) decisions which then in turn impact the lives of individuals. In that regard, accuracy is far too important to be simply pushed aside for the sake of data minimisation. And now comes the tricky part. All previous attempts to increase privacy, such as differentiated privacy, curation of training data, or downstream filtering applications, have had little success in actually improving the situation privacy-wise, while in almost all cases having negative effects on the accuracy of the produced results. A satisfying solution for this dilemma is yet to be found, however, this is not where the story ends. Memorization of content, despite its obvious benefits for accuracy and detriments for data minimization or purpose and storage limitation, causes some other, worrisome effects as well and we will mention some of them in the next chapter.
-
Data Diaries: Data Memorization and the Right to be Forgotten
We have already briefly touched upon the fact that large models also memorize a significantly larger proportion of the data they are trained on. This then makes more data vulnerable to hacker attacks. For instance, it is possible to extract personal data, such as a person’s full name, address and phone number, from the models, and large models have been training on a lot of such data. In yet another Berkley research, the researchers successfully extracted an individual person’s name, email address, phone number, fax number, and physical address. Although personal data in this case was not secret, it was shared for a specific purpose and later reproduced in a completely different context. This exemplifies a serious risk of LLMs ‘accidentally’ sharing personal data in response to queries from third persons if they simply ‘ask the right questions’. Moreover, the same research found that among 604 units of content recognized as memorized, 46 contained names of individuals with a further 32 containing some type of contact information of which 16 were private contact details. The fact that a relatively small percentage of content units recognized contained private contact information should not reassure us that the systems are ‘safe enough’. Quite the opposite. This may already be a good enough result if someone is searching for a particular individual on which the person already has some limited information and can thus perform so-called ‘targeted attacks’. On the other hand, if you are looking for just about anyone, then such information is a great start indeed as it gives you precisely the extra information needed to extract even more personal data. Particularly because it is very likely that a lot more personal data could be extracted if we ask the system better questions. On the other hand, it would be wrong to claim that the awareness of the severity of the problem is as recent as the public discussion about it. For instance, the problem of ‘model inversion’ and ‘membership inference’ attacks has been a topic of scientific papers since at least 2018 when Michael Veale, Reuben Binns and Lilian Edwards came forward with the proposition to make the entire model be considered personal data to protect the data that may be extracted from it. Their conclusion was that such an answer is straightforward since personal data can be extracted from the models using model inversion, whereas membership inference attacks can be used to determine whether an individual is included in the data set, which is in and of itself enough to be considered personal or in some cases (e.g. models trained to recognize signs of dementia or other diseases) even sensitive data. In that sense, they compared the models with pseudonymized data which is still protected under the GDPR. Although their arguments are still quite compelling and would definitely increase the role of privacy in the developmental stages of models such as GPT, their conclusion seems way too ambitious in the aftermath, with even the standard provisions of lawful, transparent and fair processing failing to be consistently and effectively applied to the training stage of these models.
Lastly, the real punch line is that, while it may be possible for the model to memorize data units contained only once in the training set, it appears the data may be memorized forever. Considering that data removed from the Internet can still be extracted from the GPT2 model is especially alarming, with the model serving as an “unintentional archive for removed data”. The effects of this on the principle of storage limitation as well as the right to be forgotten are mind boggling. More worrying still is the fact that there is no satisfactory solution for this problem. Whereas potential solutions for unlearning certain data have been proposed and developed for years, all of them fail to fully comply with the standard set by the GDPR and that for a multiplicity of reasons. Starting with the obvious fact that training large models is very expensive and the big players are not particularly motivated to retrain their models without certain data points. To the conclusion that extracting specific data points from existing models is impossible to do if the models aren’t predesigned to offer such a possibility, while even then the results are far from perfect. Thus, it seems we have a right to be forgotten by Google, but not by the large language models?
-
Conclusion
The questions raised in this article are just some of the most important ones not yet answered or (arguably) even seriously discussed in the public sphere. And these absolutely need to be tackled with, to protect the average users and consumers from the new and hardly identifiable risks of the shiny new technology helping them write more eloquent emails. LLMs such as GPT3 can be used as wonderful tools for instigating creativity or making people sound ‘posh’ even though their English level is actually not that high. However, they can also be used for something quite different (not to be overly dramatic and say evil). To turn a blind eye to the fact that these systems can be used as infinite archives of personal data since deleted or can help justify important decisions, even though they often lack accuracy in their results, would only amplify the problem, making it even harder to solve in the aftermath. How exactly this problem can be solved is open for discussion, but valid propositions, such as targeted differentiated privacy, have already been suggested. In any event, ignoring the problem and hoping it goes away is not a satisfying solution. On the other hand, it remains to be seen whether OpenAI can successfully respond to at least some public concerns, many of which were also raised in Garante’s decision temporarily suspending their service in Italy. As well as whether this decision wakes up other European data protection authorities from the winter sleep they appear to have fallen into.
1See, for example, David B. Shrestha ‘ImageNet Gets A Privacy Overhaul, What About Other Datasets?’, AIM, 22 March 2021 https://analyticsindiamag.com/imagenet-gets-a-privacy-overhaul-what-about-other-datasets/ (accessed on the 6 February 2022); Jimmy Whitaker ‘The Fall of ImageNet’, Towards Data Science, 19 March 2021 https://towardsdatascience.com/the-fall-of-imagenet-5792061e5b8a (accessed on the 6 February 2022); Khari Johnson ‘ImageNet creators find blurring faces for privacy has a ‘minimal impact on accuracy’’, VentureBeat, 16 March 2021 https://venturebeat.com/ai/imagenet-creators-find-blurring-faces-for-privacy-has-a-minimal-impact-on-accuracy/ (accessed on the 6 February 2022); Dave Gershgorn ‘A.I.’s Most Important Dataset Gets a Privacy Overhaul, a Decade Too Late’, OneZero, 19 March 2021, https://onezero.medium.com/a-i-s-most-important-dataset-gets-a-privacy-overhaul-a-decade-too-late-6bbad8c151b5 (accessed on the 6 February 2022).
2The situation is somewhat different in the USA, where at least copyright is a very hot legal topic at the moment. See, for example, GitHub Copilot litigation, ‘We’ve filed a lawsuit challenging GitHub Copilot, an AI product that relies on unprecedented opensource software piracy. Because AI needs to be fair & ethical for everyone.’, 3 of November 2022, https://githubcopilotlitigation.com/ (accessed on the 11 April 2023), Stable Diffuision litigation, ‘ We’ve filed a lawsuit challenging Stable Diffusion, a 21st century collage tool that violates the rights of artists.
Because AI needs to be fair & ethical for everyone.’, 13 January 2023, https://stablediffusionlitigation.com/ (accessed on the 11 April 2023). Moreover, ChatGPT is already blocked in China, Iran, North Korea, Russia and Italy, with Canada and Germany considering the possibility. For more, see Brandeis Marshall ‘Lost in AI, The AI takeover has arrived and it’s backfiring’, 10 April 2023, Medium, https://medium.com/@brandeismarshall/lost-in-ai-c1ef95a23947 (accessed on the 12 April 2023).
3Eric Wallace, Florian Tramèr, Matthew Jagielski, and Ariel Herbert-Voss ‘Does GPT-2 Know Your Phone Number?’, 20 December 2020, Berkley Artificial Intelligence Research, Does GPT-2 Know Your Phone Number? – The Berkeley Artificial Intelligence Research Blog (accessed on the 3 February 2023).
4See, for example, Luca Bertuzzi, ‘Italian data protection authority bans ChatGPT citing privacy violations’, 31 of March 2023, EURACTIV.com, https://www.euractiv.com/section/artificial-intelligence/news/italian-data-protection-authority-bans-chatgpt-citing-privacy-violations/ (accessed on the 11 April 2023).
5Melissa Heikkilä ‚What does GPT-3 “know” about me?’, 31 August 2022, MIT Technology Review What does GPT-3 "know" about me? | MIT Technology Review (accessed on the 3 February 2023).
6Nicholas Carlini et al. “Extracting Training Data from Large Language Models.” USENIX Security Symposium (2020) [2012.07805v2] Extracting Training Data from Large Language Models (arxiv.org) (accessed on the 3 February 2023) p.10.
7Melissa Heikkilä ‚What does GPT-3 “know” about me?’.
8Alex Hern and Dan Milmo, ‘‘I didn’t give permission’: Do AI’s backers care about data law breaches?’, 10 April 2023, https://www.theguardian.com/technology/2023/apr/10/i-didnt-give-permission-do-ais-backers-care-about-data-law-breaches (accessed on the 12 April 2023).
9https://chat.openai.com/chat (accessed on the 7 February 2023).
10Luca Bertuzzi, ‘Italian data protection authority bans ChatGPT citing privacy violations’.
11Garante per la protezione dei dati personali, Intelligenza artificiale: il Garante blocca ChatGPT. Raccolta illecita di dati personali. Assenza di sistemi per la verifica dell’età dei minori, 31 of March 2023, https://www.garanteprivacy.it/web/guest/home/docweb/-/docweb-display/docweb/9870847#english (accessed on the 11 of April 2023).
12Melissa Heikkilä ‚What does GPT-3 “know” about me?’.
13Nicholas Carlini et al. “Extracting Training Data from Large Language Models.” p.1.
14Nicholas Carlini et al. “Extracting Training Data from Large Language Models.” p.12.
15Zhuohan Li et al. “Train Large, Then Compress: Rethinking Model Size for Efficient Training and Inference of Transformers.” ArXiv abs/2002.11794 (2020) pp.6-8.
16Nicholas Carlini et al. “Extracting Training Data from Large Language Models.” p.12.
17Nicholas Carlini et al. “Extracting Training Data from Large Language Models.” p.12.
18Including even judicial decisions. See, for example, Valerio de Stefano (@valeriodeste) 2023 [Twitter] 3 February https://twitter.com/valeriodeste/status/1621288184591122432?s=46&t=aoujfWKIbPE3TErUthsxRg (accessed on the 6 February 2023). The decision is available in Spanish at https://www.diariojudicial.com/public/documentos/000/106/904/000106904.pdf (accessed on the 6 February 2023).
19See, for example, Nils Lukas et al. ‘Analyzing Leakage of Personally Identifiable Information in Language Models’ February 2023 https://www.researchgate.net/publication/367961514_Analyzing_Leakage_of_Personally_Identifiable_Information_in_Language_Models (accessed on the 6 February 2023) pp.12-13; Nicholas Carlini et al. “Extracting Training Data from Large Language Models.” pp.12-13.
20Nicholas Carlini et al. “Extracting Training Data from Large Language Models.” p.5.
21Nicholas Carlini et al. “Extracting Training Data from Large Language Models.” p.1.
22Nicholas Carlini et al. “Extracting Training Data from Large Language Models.” p.5.
23Nicholas Carlini et al. “Extracting Training Data from Large Language Models.” pp.9-10.
24Nils Lukas et al. ‘Analyzing Leakage of Personally Identifiable Information in Language Models’ p.2.
25 For more on different kinds of extraction, reconstruction and inference attacks, see Nils Lukas et al. ‘Analyzing Leakage of Personally Identifiable Information in Language Models’.
26See, for example, Nicholas Carlini et al. “Extracting Training Data from Large Language Models.” p. 13; Taylor Shin et al. “Eliciting Knowledge from Language Models Using Automatically Generated Prompts.” ArXiv abs/2010.15980 (2020) p.9.; Huseyin A. Inan et al. “Privacy Analysis in Language Models via Training Data Leakage Report.” (2021) https://arxiv.org/abs/2101.05405 (accessed on the 6 February 2023) p.2.
27Michael Veale, Reuben Binns and Lilian Edwards ‚Algorithms that remember: model inversion attacks and data protection law’ (2018) Phil.Trans. R. Soc. A376: 20180083, http://dx.doi.org/10.1098/rsta.2018.0083 (accessed on the 12 April 2023).
28Michael Veale, Reuben Binns and Lilian Edwards ‚Algorithms that remember: model inversion attacks and data protection law’ pp.6-8.
29Michael Veale, Reuben Binns and Lilian Edwards ‚Algorithms that remember: model inversion attacks and data protection law’, p.6.
30Nicholas Carlini et al. “Extracting Training Data from Large Language Models.” p.10.
31Nicholas Carlini et al. “Extracting Training Data from Large Language Models.” pp.10-11.
32Michael Veale, Reuben Binns and Lilian Edwards ‚Algorithms that remember:model inversion attacks anddata protection law’.
33See, for example, Salvatore Raieli ‘Machine unlearning: The duty of forgetting; How and why it is important to erase data point information from an AI model’ 12 September 2022, Medium, https://towardsdatascience.com/machine-unlearning-the-duty-of-forgetting-3666e5b9f6e5 (accessed on the 12 April 2023).
34 See, for example, Lucas Bourtoule et al. ‘Machine Unlearning‘ (2020) 42nd IEEE Symposium of Security and Privacy https://arxiv.org/pdf/1912.03817.pdf (accessed on the 12 April 2023).
35Nils Lukas et al. ‘Analyzing Leakage of Personally Identifiable Information in Language Models’ p.13.